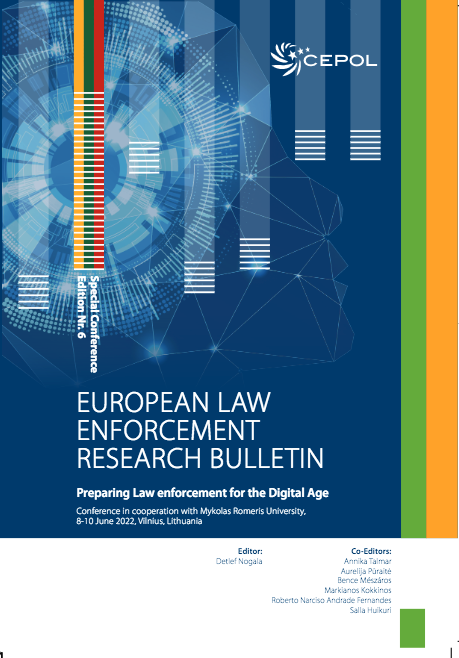
Maria Jofre
Investigating High-Risk Firms
A Machine Learning-based Approach to Cross-Border Ownership Data
Corporate ownership secrecy has become a central issue in the global debate as the use of legitimate companies in illicit schemes has increased dramatically in recent times. While several measures have been implemented worldwide to increase the transparency of firms and their owners, empirical evidence and knowledge on the subject remain limited to few small-scale case studies. In addition, there is a lack of tools specifically designed for risk assessment and risk monitoring of firms to be used by public authorities. The present paper, based on the results of the EU-funded project DATACROS, addresses these gaps by (i) proposing and validating novel risk indicators of corporate ownership opacity in a large sample of companies, and (ii) implementing them in a user-friendly platform to be used by public institutions, a tool capable of identifying companies at risk of involvement in corruption and money laundering. Machine learning results confirm the relevance of corporate ownership opacity in the facilitation of financial crime. Firms with (i) more complex structures, (ii) links to secrecy jurisdictions, and (iii) links to opaque corporate vehicles, are, in fact, more prone to engage in illicit activities. This urgently calls for the innovation of risk assessment activities based on the intelligent use of corporate ownership information. As such, the present contribution could be used to support LEAs and other authorities in combating financial crime in the sometimes overwhelming and ever-evolving digital age.